Career Tips
AI Terms That Everyone Should Know – A ChatGPT Glossary
Since Google, Microsoft, Meta, and a lot of other companies are getting into AI, it can be hard to keep up with all the new terms. You only need to look at this glossary.
ChatGPT was probably the first time you heard of AI. The OpenAI chatbot that can answer any question and help you write poems, resumes, and fusion recipes can be very helpful. People have said that ChatGPT is like autocomplete but better.
But chatbots aren’t the only thing that AI does. It’s cool to have ChatGPT do your homework or Midjourney make interesting pictures of mechs based on their country of origin, but what it could really do is completely change economies. The McKinsey Global Institute says that this area of potential could be worth $4.4 trillion a year to the world economy. This is why you will be hearing a lot more about AI.
New words are being used all over the place as people get used to a world where AI is a part of it. Here are some important AI terms you should know, whether you want to sound smart over drinks or in a job interview.
- Artificial general intelligence, or AGI: An idea that suggests a more advanced form of AI than the one we have now, one that can do things much better than humans and also learn and improve its own skills.
- AI ethics: Principles that try to keep AI from hurting people. This is done by figuring out things like how AI systems should gather data or handle bias.
- Anthropomorphism: When people tend to give nonhuman objects humanlike traits. In AI, this can mean thinking that a chatbot is more humanlike and aware than it really is, like thinking it’s happy, sad, or even sentient.
- Algorithm: A set of instructions that tells a computer program how to learn and look at data in a certain way, like finding patterns, so that it can then learn from that data and do things on its own.
- Artificial intelligence, or AI: The use of technology to simulate human intelligence, either in computer programs or robotics. A field in computer science that aims to build systems that can perform human tasks.
- Alignment: Tweaking an AI so that it does what you want it to do better. This can mean a lot of different things, from controlling content to keeping interactions with people positive.
- AI safety: An interdisciplinary field that looks at the long-term effects of AI and how it could quickly grow into a superintelligence that is hostile to humans.
- Bias: For large language models, mistakes happen because of the training data. Based on stereotypes, this can lead to wrongly attributing certain traits to certain races or groups.
- Chatbot: A program that communicates with humans through text that simulates human language.
- ChatGPT: An AI chatbot developed by OpenAI that uses large language model technology.
- Cognitive computing: Another term for artificial intelligence.
- Data augmentation: Remixing existing data or adding a more diverse set of data to train an AI.
- Deep learning: A type of AI and a subfield of machine learning that uses many factors to find complicated patterns in text, images, and sounds. The method is based on the way the brain works and makes patterns using artificial neural networks.
- Diffusion: A way for computers to learn that adds random noise to already existing data, like a picture. Diffusion models teach their networks how to fix or get back that picture.
- Emergent behavior: When an AI model exhibits unintended abilities.
- End-to-end learning, or E2E: A type of deep learning in which a model is taught how to complete a task from beginning to end. It’s not taught to do things in a certain order; instead, it learns from what it is given and solves the problem all at once.
- Ethical considerations: An awareness of the ethical implications of AI and issues related to privacy, data usage, fairness, misuse and other safety issues.
- Foom: Also known as fast takeoff or hard takeoff. The concept that if someone builds an AGI that it might already be too late to save humanity.
- Generative adversarial networks, or GANs: A generative AI model is made up of two neural networks that work together to make new data: a generator and a discriminator. The discriminator checks to see if the content is real, while the generator makes new content.
- Generative AI: A technology that makes content by using AI to make text, video, computer code, or pictures. A lot of training data is fed to the AI, which then looks for patterns to come up with its own responses, which are sometimes similar to the original data.
- Google Bard: An AI chatbot by Google that functions similarly to ChatGPT but pulls information from the current web, whereas ChatGPT is limited to data until 2021 and isn’t connected to the internet.
- Guardrails: Policies and restrictions placed on AI models to ensure data is handled responsibly and that the model doesn’t create disturbing content.
- Hallucination: An incorrect answer from AI. Can include generative AI that gives answers that are wrong but are given with confidence as if they were right. We don’t fully understand why this is happening. If you ask an AI chatbot, “When did Leonardo da Vinci paint the Mona Lisa?” it might give you the wrong answer and say, “Leonardo da Vinci painted the Mona Lisa in 1815,” which is 300 years after it was painted.
- Large language model, or LLM: An AI model trained on mass amounts of text data to understand language and generate novel content in human-like language.
- Machine learning, or ML: A part of AI that lets computers learn and make better predictions without being explicitly programmed to do so. It can be used with training sets to make new content.
- Microsoft Bing: A search engine made by Microsoft that can now use the same technology that powers ChatGPT to give you results that are powered by AI. Being connected to the internet makes it like Google Bard.
- Multimodal AI: A type of AI that can process multiple types of inputs, including text, images, videos and speech.
- Natural language processing: This area of AI uses machine learning and deep learning to help computers understand human language. It does this by using learning algorithms, statistical models, and rules for language use.
- Neural network: A computer model that looks like the structure of the human brain and is meant to find patterns in data. is made up of neurons, which are interconnected nodes that can learn and spot patterns over time.
- Overfitting: Error in machine learning where it functions too closely to the training data and may only be able to identify specific examples in said data but not new data.
- Paperclips: Nick Bostrom, a philosopher at the University of Oxford, came up with the idea of the “Paperclip Maximizer.” This is a made-up situation in which an AI system will make as many real paperclips as it can. An AI system might use up or change all materials in order to make as many paperclips as possible in order to reach its goal. This could mean taking apart other machines to make more paperclips, machines that could be useful to people. This AI system may destroy humanity in its quest to make paperclips, which wasn’t what it was meant to do.
- Parameters: Numerical values that give LLMs structure and behavior, enabling it to make predictions.
- Prompt chaining: An ability of AI to use information from previous interactions to color future responses.
- Stochastic parrot: An example of LLMs that shows the software doesn’t really understand language or the world around it, even if the output sounds very real. This phrase refers to how a parrot can copy human speech without knowing what it means.
- Style transfer: The ability to change an image’s style to fit its content, so that an AI can understand how an image looks and use that style on another image. For example, making a self-portrait in the style of Picasso out of a Rembrandt painting.
- Temperature: Parameters set to control how random a language model’s output is. A higher temperature means the model takes more risks.
- Text-to-image generation: Creating images based on textual descriptions.
- Training data: The datasets used to help AI models learn, including text, images, code or data.
- Transformer model: This is a neural network architecture and deep learning model that learns context by following links in data, like sentences or image parts. It doesn’t have to look at each word in a sentence one at a time; it can look at the whole thing and figure out what it means.
- Turing test: It checks whether a machine can act like a person. It was named after the famous mathematician and computer scientist Alan Turing. If a person can’t tell the difference between the machine’s response and that of another person, the machine passes.
- Weak AI, aka narrow AI: AI that’s focused on a particular task and can’t learn beyond its skill set. Most of today’s AI is weak AI.
- Zero-shot learning: A test where a model has to finish a task without being given the training data it needs. One example is being able to spot a lion even though you’ve only been trained to spot tigers.
Source: CNET
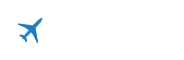